Grepr expands observability coverage by seamlessly integrating an optimized data lake into an existing environment. Observability use cases require low-latency queries and flexible schemas, capabilities that existing open-source data lake tools do not support out of the box. So how do we do it? This blog explores some of the techniques we use, specifically for logs.
1. Apache Iceberg
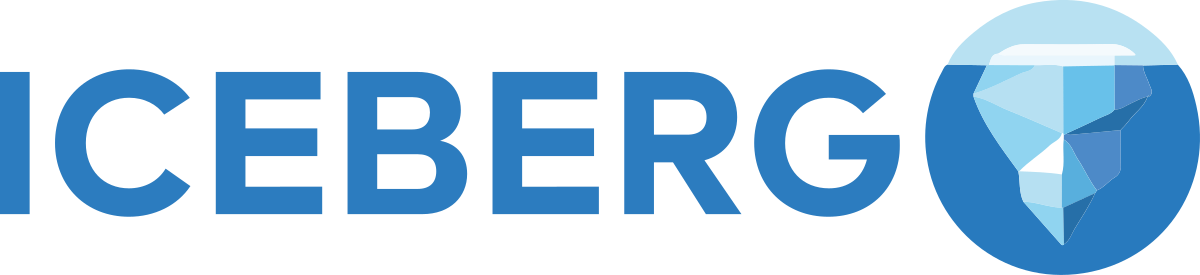
Apache Iceberg is a high-performance table format designed for large-scale data lakes. It was originally developed by Netflix to address performance and scalability limitations of older table formats like Hive. Iceberg optimizes query speed with efficient partitioning, supports schema evolution without costly rewrites, and ensures reliability with ACID transactions.
As we collect raw logs and store them into files, Iceberg also keeps track of statistics for each column of data for each file. When we need to query, Iceberg uses those statistics to filter the files that we need to read.
2. Apache Parquet for file format

Apache Parquet is a compressed columnar storage format optimized for high-performance data processing. Originally developed by Twitter and Cloudera, it is designed for efficient storage and retrieval of large-scale datasets. Parquet enables fast analytics by organizing data by columns, and maintaining statistics and dictionaries to support selective reads.
Our log files are stored using Parquet, and we make heavy use of dictionaries, bloom filters, and min/max statistics to reduce the query latency for log messages from the data lake.
3. Time-based partitioning
Partitioning by time is the most critical optimization that we do. Since most observability data (logs, metrics, events) is time-series data and all queries have a time range, partitioning by time reduces the number of data files to scan for data matching a query to only those files that match the time range.
4. Service and host partitioning
Almost all log messages are tagged by “service” and “host”, allowing us to partition the data accordingly. This partitioning accelerates searches using these tags. When Grepr summarizes logs, it includes a link in the summary message that lets users quickly access all the original raw messages. The link performs a search in our data lake using the service and host tags, with the partitioning scheme enhancing search efficiency.

5. Automatic column per tag
We use Apache Iceberg as our table format, which allows us to filter data files efficiently. By filtering based on tags, we significantly reduce the number of files that need to be scanned. However, Iceberg currently supports only equality-based filtering on column values, so enabling tag-based filtering in Iceberg requires adding a dedicated column for each tag in the schema.
This poses a challenge because Iceberg relies on a fixed schema, while tags are inherently arbitrary. To bridge this gap, Grepr automatically tracks incoming tags and dynamically creates a column for each. Managing schema updates in a distributed system like Grepr is complex, as new tags require real-time schema modifications. Grepr addresses this by coordinating schema updates with running pipelines, ensuring seamless integration of new tags.
Finally, to fully enable this functionality, our query parser ensures proper translation into SQL, leveraging the newly created columns during queries.
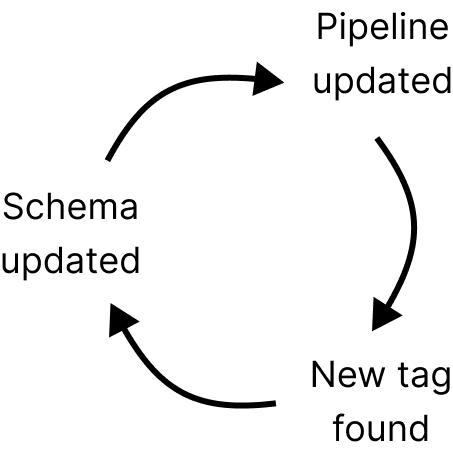
6. Right-sizing files for parallel scanning
The Grepr data lake does not use a full-text search index, which is expensive to maintain. Instead, for text queries, Grepr scans and processes log messages from selected files in a massively parallel computation. To parallelize queries as much as possible, Grepr keeps log file sizes relatively small. This increases the units of work that can be distributed to more processes and minimizes the end-to-end latency of queries.

Conclusion
This blog mentioned 6 ways we optimize the Grepr observability data lake for logs. We’re continuously looking at reducing query latency even further, without taking on more cost on ingestion. If you’d like to see what Grepr can do, get started for free here!
More blog posts
All blog posts
Using Grepr With Datadog
.jpg)
Use Grepr to Avoid Observability Vendor Lock-In
